UMRAM had broad participation in the 2024 ISMRM & ISMRT Annual Meeting & Exhibition held 04-09 May 2024 in Singapore. Two educational lectures as well as five oral, one power pitch and two digital poster presentations were delivered by UMRAM faculty and students. Educational lecture: Dr. Ergin Atalar: “Gradient Coil Design” Dr. […]
Read More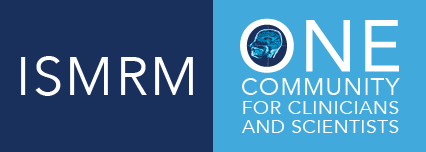